Thomas Kleine Buening (Oxford)- Strategic Interactive Decision-Making
Abstract: In many machine learning applications, algorithmic decisions impact human outcomes (e.g., loan applications, recommender systems). In response, human agents can influence these decisions by strategizing and using privileged information that algorithms rely on. This dynamic has been explored in supervised learning under the concept of strategic classification. However, strategic classification paints an incomplete picture of the strategic behavior algorithms encounter in the real world, where algorithms and agents often interact repeatedly, environments are unknown and dynamic, and agents are far-sighted and competitive. This talk argues for the importance of studying strategic interactive decision-making as a more complete perspective on the interactions and conflicts between algorithms and strategic, self-interested agents. This perspective brings together reinforcement learning and mechanism design principles to align incentives proactively and extends beyond mere strategic robustness. As a concrete example, I will present a strategic version of the contextual bandit problem, which incorporates many of the characteristics essential to strategic interactive decision-making.
Speakers
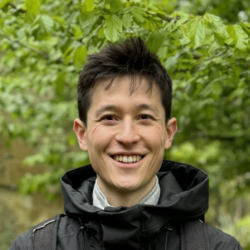
Thomas Kleine Buening
Thomas Kleine Buening is a Postdoctoral Research Associate at The Alan Turing Institute and an Associate Member of the Department of Computer Science at the University of Oxford. He earned his PhD in Computer Science from the University of Oslo. Thomas’s research focuses on reinforcement learning, with an emphasis on its intersection with algorithmic game theory and mechanism design. His work aims to address the inherently interactive and incentive-driven nature of real-world machine learning applications by designing algorithms that account for and are robust against self-interested, strategic behavior.